NCSA machine learning pipeline provides insight into energy-efficient home improvement programs
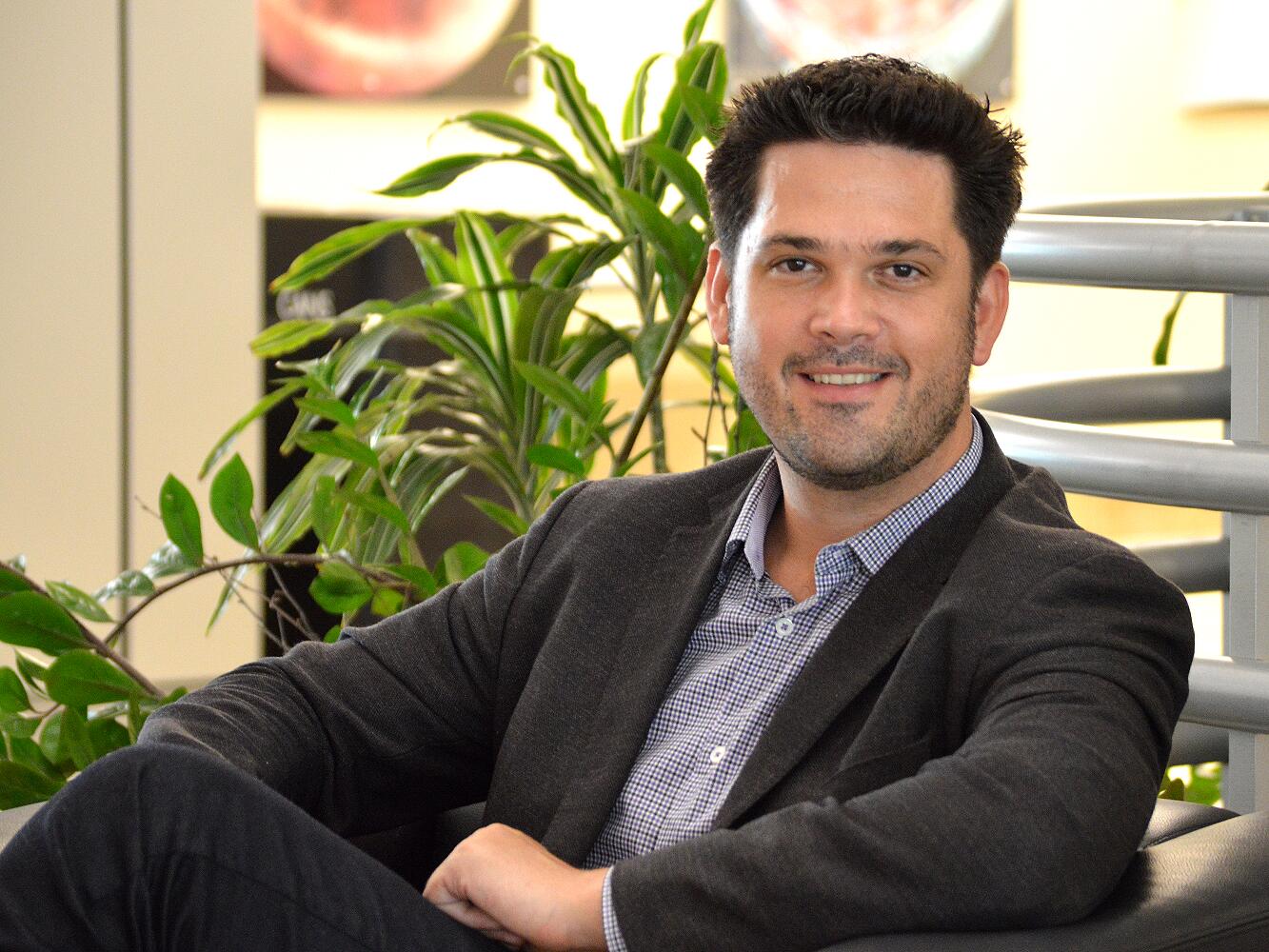
Homeowners often invest in energy-saving upgrades to make their homes more comfortable and lower their expenses, hoping to see reductions in their upcoming utility bills. But measuring the costs and effects of hundreds of different retrofits in thousands of households is a complex process.
At the University of Illinois at Urbana-Champaign’s National Center for Supercomputing Applications (NCSA), researchers are used to using compute power to dig for answers in piles of untamed data. NCSA Core Faculty member Peter Christensen, also an assistant professor of Agriculture and Consumer Economics, has developed a machine learning-driven analytics pipeline to handle data collection, pre-processing, and statistical modeling for a range of projects that involve high-dimensional data and continuous acquisition.
Over the past year, collaborators Christensen, Erica Myers and Mateus Souza of the College for Agricultural, Consumer and Environmental Sciences and Paul Francisco of the Indoor Climate Research & Training Institute have been using the BDEEP team’s pipeline and NCSA’s experimental data analytics compute cluster to analyze a large dataset of home performance measures to better understand the drivers of past savings and provide insights into how to optimize benefits in the future. Read More.