Machine learning helps researchers identify bacterial biomarkers to determine food intake
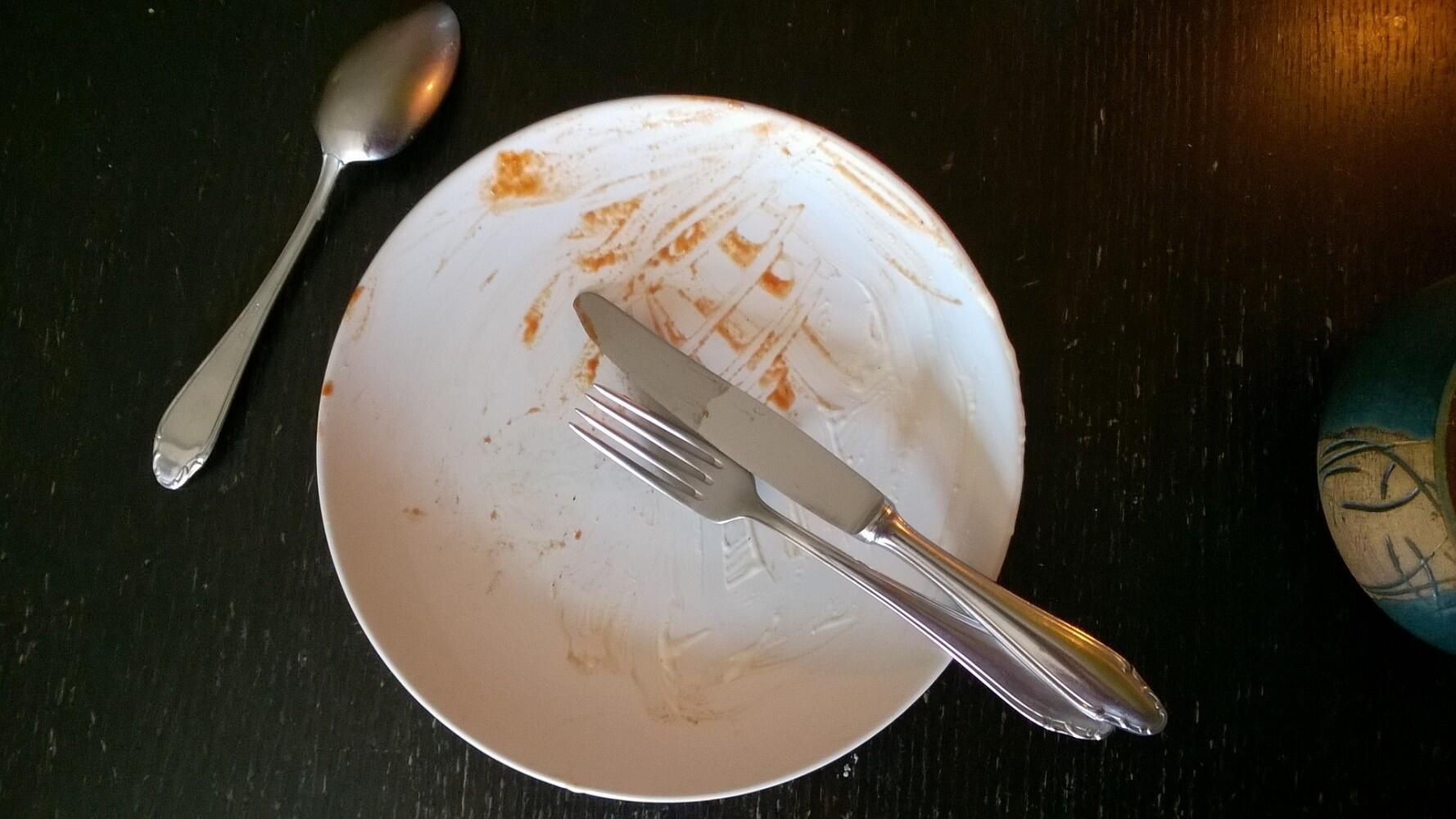
URBANA, Ill. – As food makes its way through your digestive system, gut microbiota also get energy from the food. This creates a microbial footprint of what you’ve consumed. But the detective work to find these footprints includes wrangling huge sets of data.
After all, the human gut microbiota is a collection of trillions of bacteria that reside in the gut.
A new study from University of Illinois scientists in food science and human nutrition and statistics shows fecal bacteria can be used to identify food intake with up to 85% accuracy. The scientists identified the bacterial footprints of food consumption using machine learning models and bioinformatics to study the large, complicated datasets created in microbiota studies.
Importantly, the researchers were able to narrow down the list of hundreds of bacteria to 15 to 23 unique bacteria that could differentiate between the foods consumed in the studies. Their work is a promising step in dietary biomarker research and may ultimately help inform personalized nutrition recommendations.
“The most common way to measure dietary intake is by using self-reported measures like diet records or food recalls. But the burden of recording everything you eat or trying to remember what you ate the day before reduces the accuracy of self-reported measures,” explains Leila Shinn, doctoral candidate in the Division of Nutritional Sciences (DNS) at U of I, and lead author of the study published in the Journal of Nutrition.
“Because we can quantify fecal microbes, they are a promising measure to help us identify foods that people are eating,” she adds.
Hannah Holscher, assistant professor of nutrition in the Department of Food Science and Human Nutrition at U of I, and senior author of the paper, explains the study is the first to use bacteria to identify food intake.
“Additional work is needed in the area because we're just identifying a handful of foods. But it's promising, especially with the growing interest in personalized nutrition. We could ultimately use this approach to connect the health benefits of consuming certain foods with changes in gut microbes.
“We already know that consuming walnuts, for example, reduces blood cholesterol and changes the microbiota in a certain way. Using advanced analytic approaches, like those used in our study, helps move us one step closer to personalizing nutrition advice using microbiota data. Ultimately, we envision being able to create menus to help change someone's gut microbiota in a way that will also benefit their health.”
The large dataset the researchers studied included microbiota data from participants who ate avocados, almonds, broccoli, walnuts, and whole grain oats and barley in five different feeding studies, including control and “treatment” groups for comparison. Holscher explains these foods were chosen because they can benefit health, and they contain fibers and different fatty acids that impact the gut microbiota.
The researchers sequenced the bacterial DNA from fecal samples to characterize all of the bacteria present in each participant's gut microbiota before and after they ate the study foods. Shinn and Holscher partnered with researchers in statistics at the National Center for Supercomputing Applications at U of I to use advanced analytic approaches to better analyze the “big” microbiome data.
The goal was to identify bacterial biomarkers, or objective characteristics, that could be measured in fecal samples to determine if someone has consumed a specific food or not.
“For our purposes, we wanted to narrow down the long list of candidate biomarkers to a small set of bacteria to identify which foods people had eaten,” Shinn says. “We were able to do that with a machine learning, multi-class classification model, called random forest, with up to 85% accuracy.”
The researchers went through a series of analytical “cross-checks” of the biomarker data to test the accuracy of the food predictions. “Since we went through a series of steps to validate our approach, we are pretty confident that the only bacterial signal that was left was from the food itself,” Holscher explains.
The work represents an important step in the field of dietary biomarkers.
“We're optimistic that when we integrate not just the microbes, but also their genes and their metabolites, we can improve the accuracy of our model even more. Ultimately, integrating fecal biomarkers with biomarkers from urine or blood could bolster the objective assessments of dietary intake,” Holscher says. “As the field continues to move forward, we'll be better able to make recommendations for how to modulate the microbiome for health benefits.”
The paper, “Fecal bacteria as biomarkers for predicting food intake in healthy adults," is published in the Journal of Nutrition [DOI: 10.1093/jn/nxaa285]. Authors include Leila M. Shinn, Yutong Li, Aditya Mansharamani, Loretta S. Auvil, Michael E. Welge, Colleen Bushell, Naiman A. Khan, Craig S. Charron, Janet A. Novotny, David J. Baer, Ruoqing Zhu, and Hannah D. Holscher.
The Division of Nutritional Sciences (DNS) and the Department of Food Science and Human Nutrition are in the College of Agricultural, Consumer and Environmental Sciences (ACES) at University of Illinois.
Holscher is also an affiliate of DNS. She and Zhu are also NCSA Faculty Fellows.
Funding was provided in part by the Foundation for Food and Agriculture Research New Innovator Award, USDA National Institute of Food and Agriculture, Hatch Project 1009249, ACES Jonathan Baldwin Turner Fellowship, and the National Center for Supercomputing Applications (NCSA) at the University of Illinois Urbana-Champaign through the NCSA Faculty Fellows program.